STT's MSU Foundation Professor Taps Maiti partners with ECE Professor Selin Aviyente on new NSF-funded project for statistical machine learning theory and methods with spatio-temporal data.
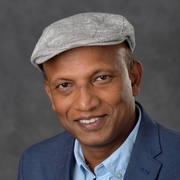

Prof. Maiti is the PI on a new award, with ECE Prof. Aviyente as co-PI, funded by the National Science Foundation’s solicitation on Algorithms for Threat Detection, a program in NSF's Division of Mathematical Sciences. This project aims at providing innovative ways of modeling spatio-temporal data, artificial neural networks, and tensor and classifying spatial objects. The proposed research builds upon capturing spatio-temporal dependence using machine learning techniques to avoid modeling large covariance matrix and capture complex spatio-temporal dependence. The planned techniques avoid specifying big covariance matrices, making the models computationally efficient and less reliant on distributional assumptions. The proposed mathematical foundations for these methods develops new statistical theories; it avoids value loss from these machine learning methods when they lack adequate mathematical justifications. The researchers will consider computer vision, as an application example which generally comes with low numbers of training samples. Popular machine learning techniques such as deep net, neural net, or higher order tensors require large training samples for building effective systems, and are thus inadequate for most vision applications. A major thrust of the project is to overcome this issue by adopting several dimension reduction techniques that can handle high-dimensional objects.